Predicting and Visualizing Overdose Risk for Public Health
2023
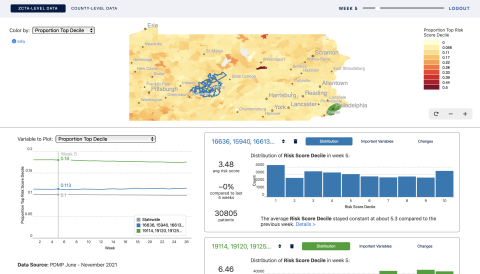
We built a visualization dashboard called Rx RiskMap that communicates and explains the results of a model to predict overdose risk across the state of Pennsylvania. The data shown is outdated and depicted for demonstration purposes only.
Overdose due to opioid misuse and abuse is currently a critical public health issue in the United States and worldwide. Machine learning (ML) approaches have been developed and deployed to help medical providers assess a patient's risk for overdose-related death before prescribing opioids. But it is unknown whether similar predictions can also be used for public health to decide where and when to allocate preventative resources at a population scale. In collaboration with the University of Pittsburgh's Center for Pharmaceutical Policy and Prescribing, we have developed and evaluated a prototype visualization system to test the feasibility of ML-driven risk visualization for local public health decision-making. To evaluate the system, we conducted a focus group study with experts from three regional health departments. These experts were receptive to the potential benefits of ML-based risk assessment, but they also expressed more fundamental needs around data visualization and were hesitant to adopt risk assessments solely based on prescription data. Based on these results, our paper discusses how public health agencies and data scientists can work towards ML-driven tools that better support people as they make these important decisions.
Researchers
Adam Perer ,
Yejun Kwak (undergraduate research assistant)
Research Areas