TouchPose Technology Senses What Touchscreens Can't Feel
News
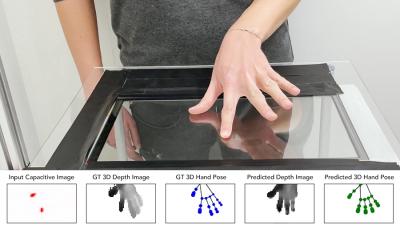
Current touchscreens and trackpads miss a lot of information.
While the technology for picking up finger interactions on these surfaces continually improves, Karan Ahuja, a doctoral student in human-computer interaction, began thinking about what else researchers might discern beyond finger contacts on a touchscreen.
"All your interactions with the screen are two-dimensional, but your hands have very complex 3D geometries," Ahuja said. "I wanted to see if you could use that knowledge of how the hand is shaped in the moment of interaction to increase the fidelity of information you are exchanging."
Ahuja worked with Paul Streli and Christian Holz in the Department of Computer Science at ETH Zürich during his residency there to develop TouchPose, a neural network estimator that calculates hand postures based on the geometry of finger touch points on smartphone and tablet touchscreens. The researchers believe it is a first-of-its-kind tool, and the team published its findings in a paper for the 2021 User Interface Software and Technology Symposium.
Research in robotics, virtual reality and other fields has provided a strong library of human hand forms and motion dynamics. Ahuja's idea determines if the hand's posture could be reverse engineered based on finger information from the screen.
For example, if you move your hand back and forth while keeping the tip of your index finger on a touchscreen, nothing happens. But if a smartphone tool could process the changing shape of your fingertip on the screen, it could infer whether your hand was moving to the left or right, forward or back, and your finger could be used like a joystick. A tool like this could also help eliminate false touch mistakes and ambiguities, which cause frustration and slow users down.
The final data set to train the model contained more than 65,000 images. To build it, Ahuja and his colleagues spent a year recording 10 participants interacting with a flat screen using 14 unique hand positions. For the model, the team developed a new machine learning architecture to handle the novel nature of the research.
"We needed to come up with some creative ways to incorporate depth data and assess the validity of touch events to produce something that could work in the wild," Ahuja said.
The researchers produced a tool that can generalize, learn and infer intent from gestures that the model had not previously seen.
"We can't know 100% what the user's hand is going to look like, so we use probable forms from the data set we captured naturally," Ahuja said. "If you have a situation where there's a single touch point, and the model can't resolve whether it's the index finger or the middle finger, it will use probabilistic understanding to figure it out."
TouchPose could enhance a touchscreen with just a simple upgrade, according to Mayank Goel, an assistant professor in the Human-Computer Interaction Institute and Ahuja's faculty adviser.
"It can unlock new capabilities with just a software upgrade and no hardware modification," Goel said.
To support the work of other researchers in the field, Ahuja and his colleagues have publicly released all their training data, code and the model itself.
"I feel that, being in academia, sharing your tools to help others do research in the domain or adjacent domains is important," Ahuja said. "We've had a lot of positive responses to the research, and we're hoping it can spark new ideas."
Related People
Karan Ahuja